Welcome to the Podiatry Arena forums
You are currently viewing our podiatry forum as a guest which gives you limited access to view all podiatry discussions and access our other features. By joining our free global community of Podiatrists and other interested foot health care professionals you will have access to post podiatry topics (answer and ask questions), communicate privately with other members, upload content, view attachments, receive a weekly email update of new discussions, access other special features. Registered users do not get displayed the advertisements in posted messages. Registration is fast, simple and absolutely free so please, join our global Podiatry community today!
-
Have you considered the Clinical Biomechanics Boot Camp Online, for taking it to the next level? See here for more.Dismiss Notice
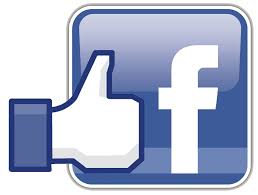

Statistics, Are they a valid test for results of clinical research
Discussion in 'General Issues and Discussion Forum' started by David Smith, Feb 26, 2008.
- Similar Threads - Statistics valid test
-
- Replies:
- 0
- Views:
- 2,989
-
- Replies:
- 1
- Views:
- 5,855
-
- Replies:
- 15
- Views:
- 12,158
-
- Replies:
- 0
- Views:
- 3,560
-
- Replies:
- 7
- Views:
- 14,334
-
- Replies:
- 5
- Views:
- 15,508
-
- Replies:
- 2
- Views:
- 10,974